“The Undervalued Hitter Study” Created by: Max Ellenbecker, Bix Ellenbecker, and Travis Ice
Data in study ranges from 2005-2019. Full study and searchable interface available on previous page.
Quick Summary
The basic purpose behind this study is to find value in hitters that is not revealed through any statistical metrics currently available. When we began, we foresaw several possible uses for this study. First and foremost, we believed that this study would reveal hitters who contribute more to playoff success than their overall performance numbers would indicate. We believe we have convincingly established this principal and identified undervalued hitters through this study. We also believed that this study could be used to identify hitters that are likely to improve on past performances or rebound from disappointing seasons and hitters that are likely to decline from past performances before it become readily apparent. We are still analyzing the data in these areas.
What we did in this study was differentiate a hitter’s performance based on the quality of pitcher they were facing, which explains why we conveniently titled them “differential hitting statistics.” Pitchers were given a rating on a 1 to 5 scale. In other words, we split up a hitter’s overall statistical performance numbers five ways so you could see how a hitter performed against each tier of pitcher individually. The ability to hit good pitching (top three tiers) is a valuable skill for a hitter to have because 95 percent of the innings pitched in the playoffs comes from these types of hitters. Additionally, we believe the ability to hit good pitching is indeed a skill; OBP and SLG correlation coefficients are at about 0.6.
Finally, this study would have no significance unless these differential statistics could predict future performance. Or in other words, unless a hitter’s past differential statistics correlated to future performance. Through a correlation study, we were able to establish that these statistics have almost identical correlation factors to conventional metrics.
Introduction
By looking at a hitter’s overall statistical numbers over the course of a single season or career you can gain a fairly accurate representation of their performance and ability most of the time, but not all of the time. That is the basic premise behind our idea. The goal of this study was to look at how individual hitters performed against pitchers of differing quality. It began with the notion that some hitters “pad” their stats by feasting on bad pitchers while other hitters perform no better, or at least not much better, regardless of whether they are facing David Price or Luke Hochevar. The latter may be a quality that remains hidden in a hitter’s overall statistical numbers.
We began by assigning a pitcher rating, from one to five, to every pitcher for each of the last seven seasons. This pitcher rating was based on a metric that included pitching stats which we felt best defined a pitcher’s ability. Then each hitter’s performance against each pitcher rating was compiled into what we termed “differential statistics”.
What we found is that most hitters follow a standard regression depending on what type of pitcher they were facing. This regression remained pretty much consistent for each of the nine years studied (see below). There were, however, several instances where hitters did not follow this trend. Some hitters tended to provide most of their contribution when facing the lower tier pitchers, falling well below the league average against the top two or three pitcher ratings. Others performed similarly no matter who they were facing. We believe these types of situations could show an underlying value in a hitter’s contribution.
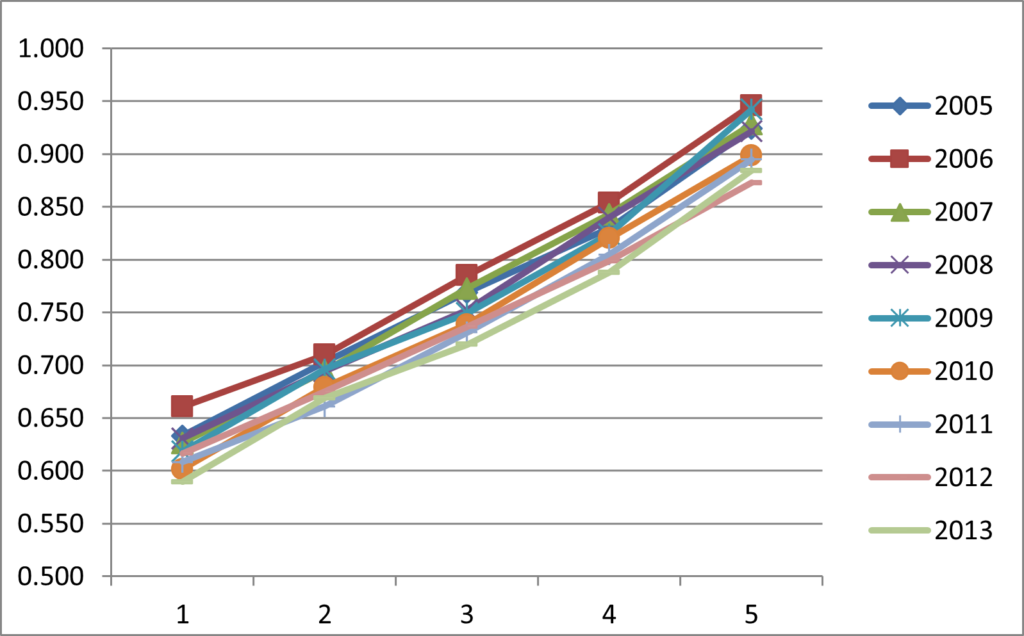
Essentially, we believe that differentiating a hitter’s offensive numbers based on the quality of pitcher faced reveals a hitter’s value that would otherwise remain hidden, as well as be an indicator of future increase or decline in performance. Hitters that perform well against better pitchers yet put up marginal numbers overall become more valuable in the playoffs because a substantial amount of innings pitched in the playoffs are by better pitchers. Identifying young hitters who have performed well against good pitching but have subpar numbers elsewhere might be an indicator of a hitter on the verge of a breakout season. Conversely, identifying hitters whose performance against the top pitchers has turned subpar may be an indicator of a hitter ready to decline.
To give you an idea for how to read and use these statistics, we have provided an example. Here are the both Dustin Pedroia’s and Ty Wigginton’s offensive statistics from 2006 to 2012.
Dustin Pedroia:
PA |
AVG |
OBP |
SLG |
OPS |
3824 |
.303 |
.369 |
.461 |
.830 |
Ty Wiggington:
PA |
AVG |
OBP |
SLG |
OPS |
3410 |
.263 |
.324 |
.443 |
.767 |
Now look at Pedroia’s and Wigginton’s offensive statistics for those same seasons except differentiated by the quality of pitcher faced.
Dustin Pedroia:
Pitcher Rating |
PA |
AVG |
OBP |
SLG |
OPS |
1 |
321 |
.260 |
.307 |
.368 |
.675 |
2 |
873 |
.277 |
.344 |
.422 |
.766 |
3 |
1410 |
.303 |
.364 |
.453 |
.817 |
4 |
934 |
.328 |
.396 |
.520 |
.916 |
5 |
286 |
.347 |
.434 |
.547 |
.981 |
Ty Wiggington:
Pitcher Rating |
PA |
AVG |
OBP |
SLG |
OPS |
1 |
295 |
.237 |
.274 |
.417 |
.691 |
2 |
759 |
.254 |
.314 |
.394 |
.708 |
3 |
1373 |
.272 |
.330 |
.477 |
.807 |
4 |
777 |
.260 |
.321 |
.432 |
.752 |
5 |
206 |
.285 |
.343 |
.473 |
.816 |
When looking only at Pedroia’s and Wigginton’s overall numbers, Pedroia is clearly superior. But, when looking at each player’s differential hitting statistics, both Pedroia and Wigginton have been very similar when facing the top three pitching tiers. Pedroia’s better overall offensive numbers are a product his superior ability to perform well against weaker pitchers. While we are not arguing that Pedroia and Wigginton are equals, we believe the differential hitting statistics show that in certain situations, both players are very similar. This becomes meaningful in the playoffs, for example, where hitters are far more likely to face pitchers from the top three pitching tiers.
Along with an explanation of the methodology used and hitter database, are two studies that aimed at identifying undervalued hitters and examining how these differential statistics affect a team’s playoff performance. The “HITTER SEARCH” tab serves as a platform for the database, which includes every hitter’s statistical performance against each pitcher rating for each of the last six seasons. It will allow you to manually enter a player’s name or select any player from the drop-down menu. All of that hitter’s statistical data over the last six years will be displayed along with their combined performance during that span. The “TEAM SEARCH” tab allows you to see each team’s performance from 2005 to 2013 against each pitcher rating. Each team’s line is a compilation of all the hitters for that team during the given year. For a perspective on how hitters and teams performed compared to the rest of the league, the league averages for each season was compiled and displayed in the “LEAGUE AVERAGES” tab.
Explanation of Methodology
This study differs from others in many regards. Existing stats are available that separate pitchers into tiers according to breakpoints in stats. For example, one can find a stat that states “Hitter X has a 0.330 average against pitchers in the top 10% of era”. This is indicating that a hitter hits .330 against a tier of pitching relative to ERA. Our study attempts to get to the root of the question, “What is a good pitcher and how hard is it to get a hit off him?” by creating an algorithm that attempts to blend a subset of statistics and subjective qualities to rank pitchers.
Anyone who has played baseball knows that stats aside, some pitchers are very hard to hit and others are not. Hitters with the ability to succeed against the best pitchers in the game give them a quality that few hitters own. We wanted to develop a metric that would determine which hitters display this ability. What we came up with was a system that would identify every pitcher into subsets based on their ability and then compile each hitter’s performance against these subsets. Before we could begin to evaluate hitters, we needed to determine a strategy for which we would rate each pitcher. The method that we decided upon utilizes a variety of stats and ratings to form a composite end result that takes into account several parameters that define a pitcher’s ability. It combines a series of statistics sorted using both bell-curve and power-law techniques and places a weight variable that is inherent in pitching. Nothing is exact, however, to steal from Warren Buffet and John Maynard Keynes, “We would rather be vaguely right, than precisely wrong.”
We are not claiming any extraordinary new method to determine the value or ability of a pitcher, we simply derived a method to incorporate statistics that define what makes a pitcher tough to hit. Our system uses similar parameters that are common in many current statistics that evaluate a pitcher’s performance today, such as the FIP method. The methodology in which we rated pitchers is then applied to a hitter’s performance in order to compile a set of what we would call “differential statistics”. We are attempting to identify hitters that are either undervalued or overvalued according to how they perform against different tiers of pitching. Several techniques, constants and proportions had to be juggled in our algorithm; however the end result did not drastically swing the outcome of expected results in most cases. Many of the results indicated what could be reasoned by simple intuition. However, there are some players that tend to stick out in both directions. Some of these players are well known and some are not. Knowledge of both sets of players offers value in the baseball marketplace.
Before any hitters could be evaluated, the first step that needed to be completed was to determine a mechanism to rate every single pitcher who threw a pitch during the past five seasons. This was accomplished by rating them on a scale from one to five (1 being the best, while 5 being the worst). Every season was treated independently in order to secure an accurate representation of a pitcher’s ability at the current time. As stated earlier, the methodology for our rating system uses similar metrics to common pitching statistics. What we aimed to do was isolate the statistics that best define a pitchers ability to get hitters out. This is where we were forced to make a few assumptions. We ultimately decided upon four different statistics: strikeouts, walks, home runs, and hits allowed. While walks and strikeouts are universally considered to be measures in which to evaluate a pitcher’s ability, home runs and hits allowed not always are. Some believe that a pitchers ability to limit home runs is simply a factor of their fly ball percentage of around 11 percent. We believe to the contrary. Several pitchers perform well above or below that mark on a consistent basis. For this reason, home runs allowed were incorporated in our rating system. Hits allowed by a pitcher are generally regarded as a component of luck but we felt that it also provided an indication of how effective a pitcher was during the course of a season. The level of importance of each of these statistics was controlled by an importance factor within our computation. Every statistic we used to was regulated on a per nine innings basis so a pitchers can be compared evenly. Baselines were set up for four the different statistics, K/9, BB/9, HR/9, and H/9. These statistics were chosen based on several factors, but were ultimately chosen based on their correlation with our definition of a good pitcher. These baselines were set at 20% thresholds for each statistic; meaning the top 20% of pitchers with the best K/9 would receive a rating of 1. Rating tables were used to compile a set of parameters for each applicable statistic. Adjustments were made for starting pitchers and which league they played in. Then each of these statistics was multiplied by the previously mentioned importance factor that was determined subjectively. Strikeouts and walks allowed were considered to be of greatest value and were assigned the largest importance factor. Again the importance factors are human generated but attempt to take into which stats need to be corrected and weighted according to the definition of pitching. The numbers were then summed together to give each pitcher a rating between 1 and 5.
After the rating system was applied to each pitcher during the last seven years, we began to compile hitters’ statistics against pitchers of each rating. We have done this for seasons 2005 through 2013. As we continue to analyze hitters, we are hoping to find correlations or trends. There are two aspects of this study that we would like to look at. The first being, individually analyzing hitters; by identifying hitters that perform well against the top pitchers and those who perform poorly against these same pitchers. We would also like to look at teams as a whole, particularly playoffs teams, and look to see if there is any correlation between a team’s performance in the playoffs and how they did against the top pitchers in the regular season. Generally, teams see better pitching in the playoffs; which leads us to believe that teams with hitters that perform well against “good pitching” will be more successful in the playoffs.
Abstract of Analysis
What we were attempting to do with this project is to show that there are in fact hitters that perform at a superior level against better pitching than other hitters. We wanted to expand on the notion that certain particular players hit quality pitching better than most other hitters. This certain quality that these types of hitters have may not always show up in their overall stat line. For example, a hitter may produce an OPS of 0.800 overall, but that hitter has an OPS of 0.800 versus all types of pitchers. Whereas most hitters follow the standard league average curve and produce an OPS of 0.600 against the best pitchers in the league and an OPS of 1.000 against inferior pitching; causing their overall stat line to have an OPS of 0.800. To show that this case does in fact exist, we had to come up with a way to compare every player to the rest of the league. We decided that looking at a hitter’s OPS compared to the league average OPS versus each level of pitching quality would be the best way to do this.
To verify the consistency of our method of determining pitcher ratings, we looked at the league averages of a hitter’s stat line for each year of compiled data. This was done to ensure that a hitter’s performance, on average, would differ based on the type of pitcher they were facing. It would also verify whether there was a correlation between the league’s performance and different tiers of pitchers from year to year. This was done compiling every hitter’s stat line against each different pitcher type for each year. The following are the league averages versus each pitching tier:
2006:
Pitcher Rating |
OPS |
1 |
.661 |
2 |
.710 |
3 |
.785 |
4 |
.854 |
5 |
.946 |
2007:
Pitcher Rating |
OPS |
1 |
.627 |
2 |
.695 |
3 |
.773 |
4 |
.843 |
5 |
.928 |
2008:
Pitcher Rating |
OPS |
1 |
.631 |
2 |
.695 |
3 |
.752 |
4 |
.840 |
5 |
.922 |
2009:
Pitcher Rating |
OPS |
1 |
.618 |
2 |
.696 |
3 |
.749 |
4 |
.824 |
5 |
.942 |
2010:
Pitcher Rating |
OPS |
1 |
.602 |
2 |
.679 |
3 |
.760 |
4 |
.835 |
5 |
.928 |

Through a quick analysis of the league average performance, there is only a slight variance from year to year of a hitter’s OPS against the different levels of pitching tiers, and that as a whole the league follows a general curve between OPS and pitcher rating. This verified that in general, hitters perform better against lesser quality pitching along a standard regression. Because there was such similarity from year to year in terms of the league average performance versus each pitcher rating, we felt that using an average of the five years would give the most consistent results when analyzing hitters over the course of their career. As you can assume, attempting to really place judgment on a hitters performance over one year can lead to misleading data because the sample sizes against pitchers with a number one rating can range anywhere from thirty to sixty for everyday players. For this reason, multi-year studies will yield more consistent results; even just a two year span provides much more consistent data. We then attempted to compile a list of the top major league hitters over the past five years. Every hitter’s performance against each tier of pitcher was compiled from 2006 to 2010. The league average OPS against each pitcher rating for that five year span was used as a baseline to compare each hitter. This gave us a reference to compare each hitter’s performance to. They were as follows:
Pitcher Rating |
League Average OPS |
1 |
.628 |
2 |
.694 |
3 |
.760 |
4 |
.835 |
5 |
.924 |
In essence, we were attempting to calculate each hitters OPS above the league average. This study places an emphasis on hitters that perform in a superior manner against the better pitchers in the league. For this reason, for this reason we subtracted the league average OPS from each hitter’s OPS versus each pitcher type. Then the differential OPS against each pitcher rating was added together for each hitter. To clarify, assume that a hitter performed exactly in line with the league averages versus each pitcher rating: that hitter would have a “combined OPS above league average” of 0.000. As a filter, we limited our search to hitters that have compiled at least 150 plate appearances against pitchers with a one rating to prevent small sample sizes from skewing the results. The following list is the top performers against pitchers with either a one or two pitcher rating:
Rank |
Player |
Combined OPS Above League Average |
1 |
Albert Pujols |
.498 |
2 |
Chipper Jones |
.425 |
3 |
Ryan Braun |
.400 |
4 |
Miguel Cabrera |
.395 |
5 |
Prince Fielder |
.387 |
6 |
Alex Rodriguez |
.382 |
7 |
Lance Berkman |
.371 |
8 |
Carlos Beltran |
.369 |
9 |
Joe Mauer |
.360 |
10 |
Matt Holliday |
.345 |
11 |
Manny Ramirez |
.333 |
12 |
Joey Votto |
.328 |
13 |
Ryan Howard |
.322 |
14 |
Brian McCann |
.322 |
15 |
Jim Thome |
.287 |
This list is compiled of players that generally would be considered some of the top hitters over the last five years. It follows a general line of reasoning that the best hitters usually perform better against each type of pitcher in comparison to the rest of the league. That is not a trend that we were attempting to find because we regarded it as true in most cases. What we were attempting to find with this study is whether there are hitters that perform well above the league average versus number one and two type pitchers even though their overall stat line may not be indicative of that. We also believed that there are actually hitters who compile impressive numbers as a whole, but when broken down into stats against pitchers of differing quality, most of their production came against lesser quality pitchers and did not follow the league average differentiation between 1,2,3,4, and 5 pitcher types. We found both hypotheses to be true for several hitters. For example, look at the comparison between two hitters, Robinson Cano and Ty Wigginton. There overall stat-lines from 2006 to 2010 are very different with Robinson Cano’s being much better.
Player |
AVG |
OBP |
SLG |
OPS |
Robinson Cano |
.311 |
.351 |
.495 |
.846 |
Ty Wiggington |
.270 |
.326 |
.456 |
.783 |
Both hitters perform above the league average for this five year span in terms of their overall statistics, but Cano’s OPS is just over sixty points higher than Wigginton’s OPS. When you break down both of these stat-lines in terms of differential statistics through each pitcher rating, each Cano’s and Wigginton’s line looks very different.
Robinson Cano:
Pitcher Rating |
AVG |
OBP |
SLG |
OPS |
1 |
.217 |
.251 |
.338 |
.589 |
2 |
.285 |
.329 |
.398 |
.727 |
3 |
.310 |
.342 |
.508 |
.850 |
4 |
.342 |
.380 |
.569 |
.949 |
5 |
.407 |
.451 |
.663 |
1.114 |
Ty Wiggington:
Pitcher Rating |
AVG |
OBP |
SLG |
OPS |
1 |
.254 |
.284 |
.459 |
.744 |
2 |
.264 |
.316 |
.415 |
.731 |
3 |
.272 |
.324 |
.476 |
.774 |
4 |
.274 |
.332 |
.459 |
.791 |
5 |
.291 |
.333 |
.474 |
.809 |
Robinson Cano follows more or less the league average regression curve for each pitcher rating, although his is a little more extreme; below the league average against pitchers with a one rating and above the league average against pitchers with a four or five rating. Meanwhile, Ty Wigginton’s stat-line does not correlate with the rest of the league’s hitters. His OPS increase only slightly from a one pitcher rating to a five pitcher rating. He performs well above the league average against number one and two type pitchers, is around the league average against number three type pitchers, and below the league average against number four and five pitchers. Wigginton actually outperformed Cano when facing number one and two rated pitchers; Cano then much better numbers when facing number three, four, and five rated pitchers.
This type of situation was exactly what our study was attempting to find; hitters that don’t follow the standard regression that the rest of the league does. In these cases, that hitter generally tends to perform superior to the rest of the league against the top two pitcher ratings. This does not appear when looking at a player’s overall statistics. Yet, this could be valuable in determining the quality of a hitter’s performance. Ty Wigginton’s offensive contribution may be undervalued. He merits a skill that few hitters have; producing against the better pitchers in the game. Hitters that perform at the same level versus number one and two rated pitchers are viewed as some of the best hitters in the game, and some of the most expensive. Most would not consider Wigginton to be anywhere near that level. While he does not perform at the same level as players such as Albert Pujols, Miguel Cabrera, or Alex Rodriguez, players hit perform well above average against all levels of pitching, he is more similar than it would appear. Examining his differential stat line can be a method to expose this superior performance.
–Excel file of the full study is available for download in the ‘Undervalued Hitter Study’ portion of the website.